Face recognition software compares a photo of you with a database of public photos or existing records. The technology can be used for log-in on apps, to authenticate identity and verify passwords.
But the software isn’t always accurate. And that can lead to violations of human rights. It can lead to disproportionate scrutiny and racial profiling, for example. It can also have a chilling effect on free speech and legal dissent.
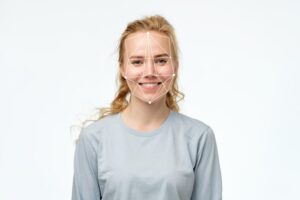
Capture
You probably think of facial recognition as a technology used by law enforcement. Police officers use it to identify fugitives and criminals from photos. The software compares the suspect’s photo with images stored in a database of faces to try to find the right match.
The software first analyzes the image to look for facial features that are unique and distinctive. It then converts those data points into a mathematical representation of your face. This is called a facial template, or a faceprint.
This digital signature is then compared with faceprints in a database to find the best match. This process can happen in real-time or asynchronously. If a match is found, the software will alert the user. For example, if you are the owner of a lost dog, an app like FindingRover will scan local shelters for pictures of your pet and report back if it finds a match. This is a great way to help reunite lost pets with their owners.
Analysis
Facial recognition software can pick out your face from a crowd, extract it and compare it to a database of images. It can also calculate accuracy scores and provide alternatives to a match.
Once the facial information is captured, it’s analyzed by a facial recognition algorithm using features like nodal points (specific coordinates marking important parts of a face, such as the width of the nose and widow’s peak). This turns your face into a digital signature, which is then searched against a database.
The search process is done using mathematical algorithms that take unique facial data into account, including bone structure, skin tone and color, pixelation, illumination, poses and other factors. These algorithms are so precise that even a small change in appearance, such as wearing cosmetic makeup or growing a beard, will not fool the system. That’s how Apple’s Face ID can recognize you even when you wear a mask. It works even in low-light conditions or with varying poses or lighting.
Matching
During this step, the facial recognition software compares your analogue information—the space between your eyes and the width of your nose, for example—to digital information—the nodal points that make up your faceprint. This faceprint is then compared to existing information in the database to see if it’s a match.
Whether it’s on Facebook or at an airport, this is the magic that allows facial recognition to link faces to profiles. Once a profile has been recognized, the technology is used to track movements and verify identities.
Attendance tracking is a time-consuming manual process for many institutions, and the facial recognition technology can speed things up, especially for remote students or those who aren’t able to come into the classroom. However, some people worry that facial recognition may allow for a form of mass surveillance that could restrict individual freedoms. It isn’t foolproof, and even a slight change in facial features or a new hairstyle could lead to someone being implicated for crimes they didn’t commit.
False negatives
Facial recognition is a powerful tool that can be used to identify people, for authentication of individuals (logins on applications and systems), and for law enforcement. However, the technology is not perfect and can be prone to bias. This can lead to a variety of adverse consequences, from a minor inconvenience in access control, to false accusations and even wrongful arrests.
One of the key factors in a facial recognition system’s accuracy is its False Negative Rate – the number of matches that are not acted upon because they were not recognised as legitimate by the software. Allevate’s experience shows that a high level of accuracy is achievable when the systems are tuned to minimise False Positives and optimise their False Negative Rate.
However, there are other factors that contribute to the accuracy of a facial recognition system that can be used by police, such as demographic differentials. For example, NIST’s testing of face recognition algorithms found that they were 10 to 100 times more likely to pick the wrong image for dark-skinned women when performing one-to-many matching.