Various Approaches Have Been Taken To Utilizing Facial Recognition And AI Technology To Improve Biometric Security, Including Face Detection And Keystroke Dynamics. EDNA And Behavioral Biometrics Are Also A Part Of The Mix. Some Countries Have Already Adopted Various Approaches For These Technologies, While Others Are Still Deciding On Their Own Course Of Action. Regardless Of The Final Outcome, However, The Debate Will Continue To Rage, As The Future Of Biometric Security Will Require Careful Consideration Of The Ethical And Legal Implications Of Such Technologies.
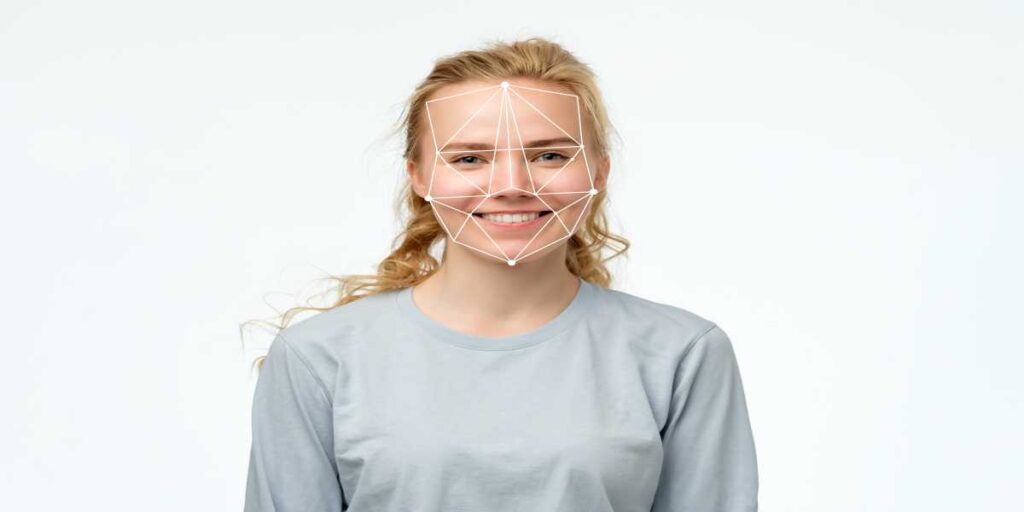
Face detection
While passwords are still the most common method of authentication, they are a rudimentary form of protection. People often create weak passwords and forget them, making it more difficult for companies to prevent fraudulent activities. Face detection using AI technology is one way to combat these problems. The advancements in AI technology are helping companies make better use of their data and increase the security of their systems. To learn more about how AI technology can improve security, read on.
The first step in the pipeline is face detection, which is the process of finding a face in an image, without concern for determining the identity of the person. Most face detection programs use a model called the Ultralight detector, which provides excellent performance when detecting faces in a variety of angles. The model is also lightweight, only 2MB in size, and very fast. This means that people with autism and other similar conditions can benefit from face detection.
Keystroke dynamics
Keystroke dynamics and AI technology in biometrics have been deemed a promising candidate for security applications. The keystroke dynamics software can integrate seamlessly into existing security systems and work environments without the need for additional hardware. It is also noninvasive and scalable. However, its use is not without its drawbacks. The MIT researchers identified that keystroke dynamics may not be as accurate as fingerprints or voice recognition, since typing patterns can vary wildly, depending on a person’s mood and the type of keyboard they use.
For biometrics to work properly, keystroke dynamics must be accurate. This requires a system to detect inconsistencies in user input and typing patterns. For example, a keystroke recognition device must record a user’s keystroke patterns for at least 15 consecutive days. Inconsistencies in the typing pattern can occur due to user errors and backspaces. The keystroke dynamics software must have a very high degree of accuracy to guarantee security.
Behavioral biometrics
Behavioral biometrics use the human behavioral characteristics to authenticate users, eliminating many of the shortcomings and concerns associated with traditional biometrics. These unique behaviors are encoded into a database and digitally sampled during authentication and verification. This is a great help for organizations who want to scale their authentication processes and to protect sensitive data. Behavioral biometrics are rapidly gaining acceptance and applications in the workplace, in e-commerce, payments, and access control.
Behavioral biometrics use a person’s physical movements to authenticate users. They have already been used in retail stores to differentiate legitimate consumers from fraudsters. These technologies have also proven effective in preventing identity theft. Further, they will continue to improve as new advances in AI technology are made. Behavioral biometrics and AI technology are a powerful combination for ensuring secure and convenient authentication of users.
eDNA
eDNA, which stands for “electronic DNA,” is a biometric authentication technology. This new technology is similar to facial recognition but concentrates on one feature of the face – the eye. It uses much less complexity than facial recognition systems. In fact, eDNA’s scanning capability can be as far away as 40 feet. The technology uses infrared sensors to detect changes to the iris. In addition, the technology can work in low-light conditions.
This technique is based on OpenCV, a facial recognition library that parses a photo and maps 64 landmarks. Then, the system feeds this cutout face image into a deep neural network (DNN) trained on the TensorFlow library. The eDNA feature vector is then generated, which contains the major biometric characteristics of a person’s face. It is 2048 bits long, and can compare with a record stored in the system database. Its resulting feature vector cannot be reverse-engineered, ensuring that potential hackers cannot get access to the original record.
Unsupervised machine learning
The use of unsupervised machine learning algorithms in biometric security has several applications. Unsupervised algorithms focus on discovering latent factors and other features, such as clusters and matrix completion. In contrast to supervised learning, which focuses on classification and regression, unsupervised algorithms focus on data protection and biometric data extraction. This ensures more accurate classification and localization of proofs. Various applications of unsupervised machine learning in biometric security are discussed in this article.
One example of using unsupervised machine learning in biometric security is to build recommendation engines based on purchase behavior data. Unsupervised learning algorithms can also help retailers make more targeted cross-selling recommendations during checkout. Unsupervised machine learning is especially useful in cybersecurity, digital art, and fraud detection. You can learn a lot about the data you’re analyzing by combining all the data. The benefits of unsupervised machine learning are numerous.
BeCAPTCHA
The developers of BeCAPTCHA have rewritten the rules of the CAPTCHA challenge by using artificial intelligence (AI) technology. Their software combines behavioral and image biometrics. This enables the system to use a person’s unique facial features to verify a response. This eliminates annoying tests of a person’s humanity. In the coming years, this technology may even make CAPTCHA challenges a thing of the past.
In this modern world, the use of biometrics is increasingly common. From consumer-facing applications to law enforcement applications, iris scanning has become an important technology. The Samsung Galaxy S9 and Note 9 smartphones use this technology to protect their systems. In addition to protecting a company’s assets, biometrics can ensure employee competency. If employees are properly trained, they can be trusted to use their devices safely and securely.